Whelp SLM vs. OpenAI's GPT: A Tale of Two Language Models
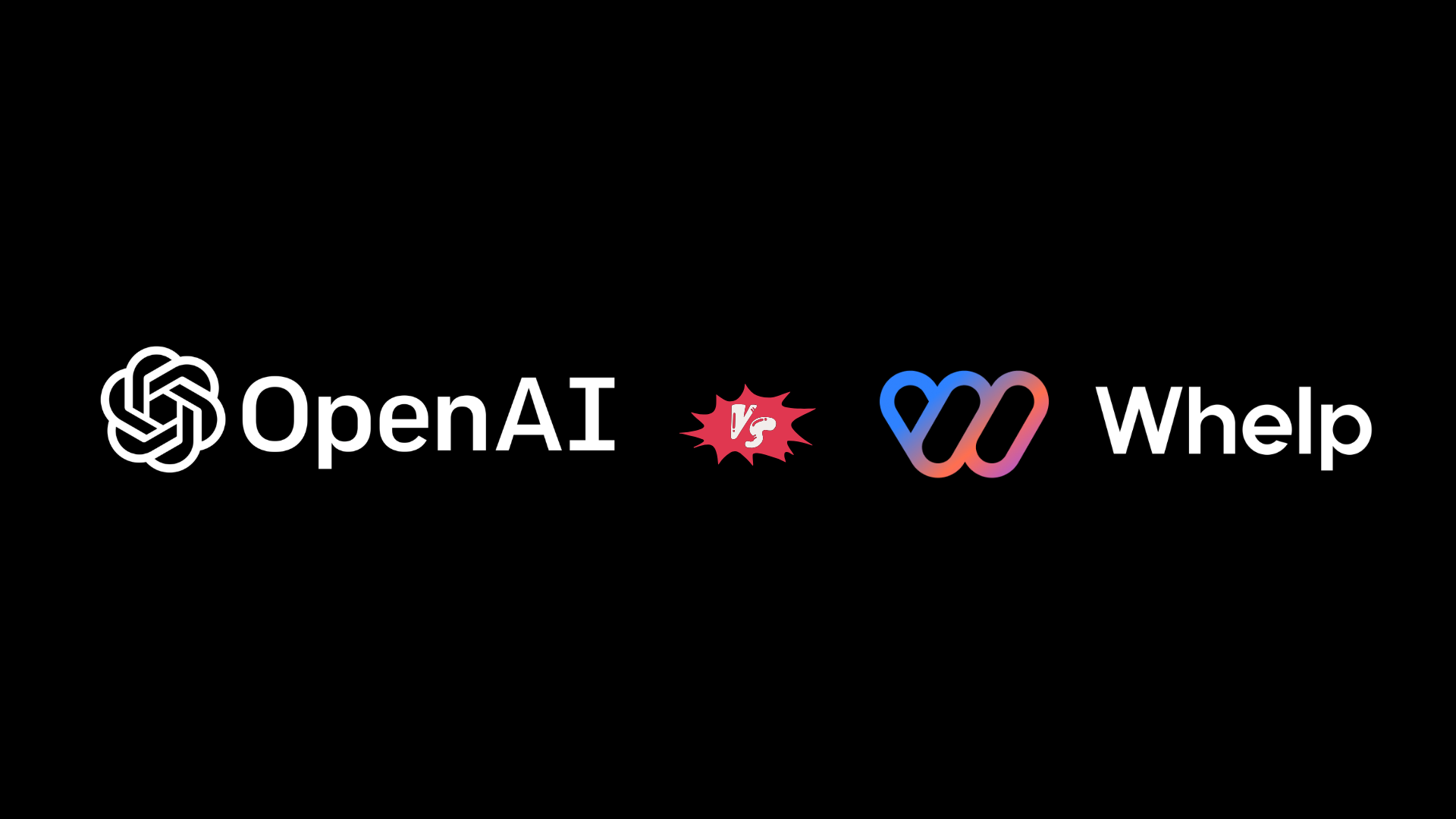
We built Whelp AI, and many people ask us what the main difference between us and other players, specially OpenAI's GPT is. The world of language models (LMs) is rapidly evolving, with new players and approaches emerging constantly. Two prominent examples, Whelp SLM and OpenAI's GPT, represent distinct philosophies in LM development. While both aim to generate human-like text, their underlying architectures and target applications differ significantly. This post explores the key differentiators between these two powerful technologies, going beyond the often-cited difference in knowledge base focus.
The most commonly discussed distinction is the scope of their knowledge. Whelp SLM (Specialized Language Model) is designed to excel within specific, narrowly defined domains. Think of a legal assistant trained on case law, a medical chatbot knowledgeable about specific diseases, or a technical support agent versed in a particular product line. SLMs are trained on curated datasets relevant to their niche, allowing them to provide highly accurate and relevant information within their area of expertise.
OpenAI's GPT (Generative Pre-trained Transformer), on the other hand, leverages massive and diverse datasets from the broader internet. This allows GPT models to handle a wide range of tasks and generate text on a multitude of topics. They possess a broader understanding of language and can engage in more general conversations. However, this breadth can sometimes come at the cost of accuracy and specificity when dealing with niche subjects.
Beyond the knowledge base, here are three more key differentiators between Whelp SLM and OpenAI's GPT:
Training Data and Methodology: GPT models are typically trained using a self-supervised learning approach on vast amounts of unlabeled text data. This allows them to learn statistical relationships between words and phrases, enabling them to generate coherent and contextually relevant text. Whelp SLM, while potentially leveraging some pre-training, often relies on smaller, highly curated datasets and may incorporate supervised learning techniques to fine-tune its performance for specific tasks. This focused training allows SLMs to optimize for accuracy and reliability within their domain.
Performance Metrics and Evaluation: Evaluating the performance of LMs is a complex task. For GPT models, common metrics include perplexity (measuring how well the model predicts the next word in a sequence) and human evaluation of coherence and fluency. While these metrics are also relevant for SLMs, the emphasis shifts towards accuracy and domain-specific relevance. For example, a medical SLM might be evaluated based on its ability to correctly answer medical questions and provide accurate diagnoses (within its defined scope). The cost of an incorrect answer is also much higher in specialized domains, making accuracy paramount for SLMs.
Deployment and Accessibility: GPT models are often accessed through APIs and platforms, making them readily available for developers to integrate into various applications. Whelp SLMs, due to their specialized nature, may require more tailored deployment strategies. They may be integrated directly into specific systems or applications within a particular organization or industry. Furthermore, the data used to train an SLM might be proprietary or sensitive, limiting its public accessibility. This controlled access allows for greater data security and control over the model's output.
In summary, Whelp SLM and OpenAI's GPT represent two different ends of the language model spectrum. GPT models are generalists, capable of handling a wide range of tasks and topics with impressive fluency.
Whelp SLMs are specialists, optimized for accuracy and reliability within specific domains. The choice between the two depends heavily on the specific application and the required level of domain expertise. As the field continues to evolve, we can expect to see even more specialized LMs emerge, catering to the diverse needs of various industries and applications.